PGMODAYS 2025
Mardi 18 novembre & Mercredi 19 novembre
EDF Lab Paris-Saclay, Palaiseau, France
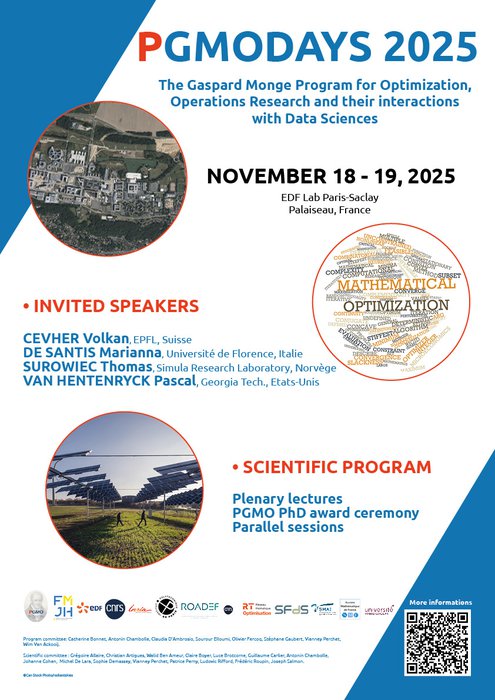
Invited Speakers
-
CEVHER Volkan (EPFL, Suisse)
-
DE SANTIS Marianna (Université de Florence, Italie)
-
SUROWIEC Thomas (Simula Research Laboratory, Norvège)
-
VAN HENTENRYCK Pascal (Georgia Tech., Etats-Unis)
Abstracts Submissions PGMODAYS 2025
Submissions on all aspects of optimization and operations research, theoretical or applied and their interfaces with data sciences are welcome.
A typical invited session consists of one block of 3 talks of 30 minutes each. Longer invited sessions with 6 talks may also be considered if the topic justifies it.
The topics of this conference include :
- continuous optimization (convex and non-convex, non-smouth...)
- optimization of large systems (decomposition-coordination methods,...)
- combinatorial optimization, integer or mixed programming
- optimization under uncertainty (stochastic and robust optimization)
- global optimization (relaxation, approximation, semi-algebraic programing, stochastic algorithms...)
- optimal control
- PDE aspects of optimization (shape optimization, optimal transport,...)
- game theory
- interfaces of optimization and data sciences, including statistical learning
- related fields : risk management, uncertainties analysis
- specific industrial applications of optimization and operations research: energy management, transportation, telecom networks,...
The leaders of PGMO projects are especially encouraged to propose presentations on the topics of their project.
Researchers willing to organize invited sessions should inform the pgmo board for pre-approval and coordination, by writing to pgmodays-coordination@fondation-hadamard.fr. After pre-apporval, they should submit via easychair a one page file with the title of the session and the list of speakers + titles.
Speakers of invited sessions should follow the same abstract submission procedure as for ordinary submissions, mentioning in addition the title of the invited session in the online form.
Deadline of abstracts submission (both for contributed talks and invited sessions) --> September 12th, 2025
Notification of acceptance --> Second half of october, 2025
Abstracts of one page should be submitted via easychair.
Please follow the guidelines and template to prepare your abstracts.